Pandas: Count like a stack using rolling
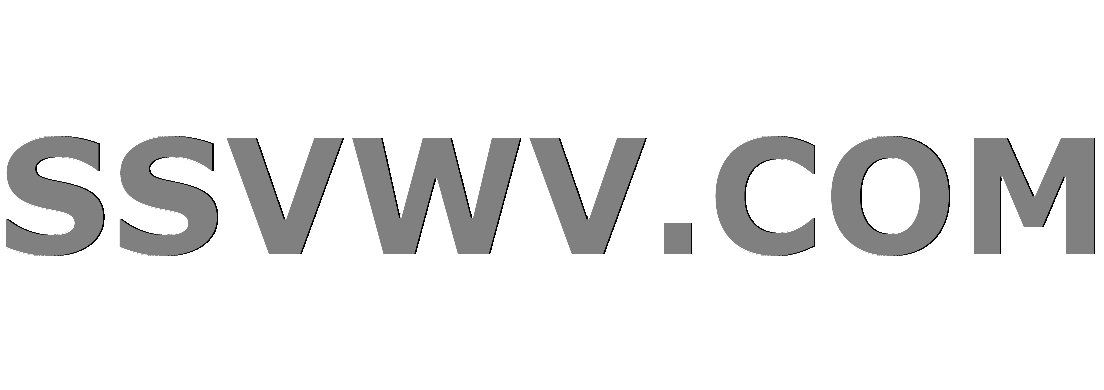
Multi tool use
I have a table like this (email is simplified to just one letter here):
timestamp | email
2018-10-17 13:00:00+00:00 | m
2018-10-17 13:00:00+00:00 | m
2018-10-17 13:00:10+00:00 |
2018-10-17 13:00:10+00:00 | v
2018-10-17 13:00:30+00:00 |
2018-10-17 13:00:30+00:00 | c
2018-10-17 13:00:50+00:00 | p
2018-10-17 13:01:00+00:00 |
2018-10-17 13:01:00+00:00 | m
2018-10-17 13:01:00+00:00 | s
2018-10-17 13:01:00+00:00 | b
Now, I would like to make a new column, that would count the number of times that the email repeated in the last 30 seconds before the entry, for example.
timestamp | email | count | comment
2018-10-17 13:00:00+00:00 | m | 1 |
2018-10-17 13:00:00+00:00 | m | 2 | (there were 2 entries in the last 30s)
2018-10-17 13:00:10+00:00 | | 1 | (empty we count as well)
2018-10-17 13:00:10+00:00 | v | 1 |
2018-10-17 13:00:30+00:00 | | 2 | (counting the empty like emails)
2018-10-17 13:00:30+00:00 | c | 1 |
2018-10-17 13:00:50+00:00 | p | 1 |
2018-10-17 13:01:00+00:00 | | 2 | (in the last 30s from this ts, we have 2)
2018-10-17 13:01:00+00:00 | m | 1 | (the first 2 m happened before the last 30s)
2018-10-17 13:01:00+00:00 | s | 1 |
2018-10-17 13:01:00+00:00 | b | 1 |
The timestamp is a dateTime object
timestamp datetime64[ns, UTC]
Also, it's the index and it's sorted.
I first tried, this command:
df['email'].groupby(df.email).rolling('120s').count().values
But it doesn't work with a string, so I converted it to a unique number, using:
full_df['email'].factorize()
But the results doesn't appear to be right:
timestamp | email | count | comment
2018-10-17 13:00:00+00:00 | m | 1 |
2018-10-17 13:00:00+00:00 | m | 2 |
2018-10-17 13:00:10+00:00 | | 1 |
2018-10-17 13:00:10+00:00 | v | 2 | (No ideia about this result)
2018-10-17 13:00:30+00:00 | | 3 | (Appears to just keeping count)
2018-10-17 13:00:30+00:00 | c | 1 | (Then just go back to 1 again... )
2018-10-17 13:00:50+00:00 | p | 2 |
2018-10-17 13:01:00+00:00 | | 3 |
2018-10-17 13:01:00+00:00 | m | 4 |
2018-10-17 13:01:00+00:00 | s | 1 |
2018-10-17 13:01:00+00:00 | b | 1 |
Any ideia what I'm doing wrong and how can I get what I would like to get?
Many Thanks,
Joao
python pandas jupyter
add a comment |
I have a table like this (email is simplified to just one letter here):
timestamp | email
2018-10-17 13:00:00+00:00 | m
2018-10-17 13:00:00+00:00 | m
2018-10-17 13:00:10+00:00 |
2018-10-17 13:00:10+00:00 | v
2018-10-17 13:00:30+00:00 |
2018-10-17 13:00:30+00:00 | c
2018-10-17 13:00:50+00:00 | p
2018-10-17 13:01:00+00:00 |
2018-10-17 13:01:00+00:00 | m
2018-10-17 13:01:00+00:00 | s
2018-10-17 13:01:00+00:00 | b
Now, I would like to make a new column, that would count the number of times that the email repeated in the last 30 seconds before the entry, for example.
timestamp | email | count | comment
2018-10-17 13:00:00+00:00 | m | 1 |
2018-10-17 13:00:00+00:00 | m | 2 | (there were 2 entries in the last 30s)
2018-10-17 13:00:10+00:00 | | 1 | (empty we count as well)
2018-10-17 13:00:10+00:00 | v | 1 |
2018-10-17 13:00:30+00:00 | | 2 | (counting the empty like emails)
2018-10-17 13:00:30+00:00 | c | 1 |
2018-10-17 13:00:50+00:00 | p | 1 |
2018-10-17 13:01:00+00:00 | | 2 | (in the last 30s from this ts, we have 2)
2018-10-17 13:01:00+00:00 | m | 1 | (the first 2 m happened before the last 30s)
2018-10-17 13:01:00+00:00 | s | 1 |
2018-10-17 13:01:00+00:00 | b | 1 |
The timestamp is a dateTime object
timestamp datetime64[ns, UTC]
Also, it's the index and it's sorted.
I first tried, this command:
df['email'].groupby(df.email).rolling('120s').count().values
But it doesn't work with a string, so I converted it to a unique number, using:
full_df['email'].factorize()
But the results doesn't appear to be right:
timestamp | email | count | comment
2018-10-17 13:00:00+00:00 | m | 1 |
2018-10-17 13:00:00+00:00 | m | 2 |
2018-10-17 13:00:10+00:00 | | 1 |
2018-10-17 13:00:10+00:00 | v | 2 | (No ideia about this result)
2018-10-17 13:00:30+00:00 | | 3 | (Appears to just keeping count)
2018-10-17 13:00:30+00:00 | c | 1 | (Then just go back to 1 again... )
2018-10-17 13:00:50+00:00 | p | 2 |
2018-10-17 13:01:00+00:00 | | 3 |
2018-10-17 13:01:00+00:00 | m | 4 |
2018-10-17 13:01:00+00:00 | s | 1 |
2018-10-17 13:01:00+00:00 | b | 1 |
Any ideia what I'm doing wrong and how can I get what I would like to get?
Many Thanks,
Joao
python pandas jupyter
add a comment |
I have a table like this (email is simplified to just one letter here):
timestamp | email
2018-10-17 13:00:00+00:00 | m
2018-10-17 13:00:00+00:00 | m
2018-10-17 13:00:10+00:00 |
2018-10-17 13:00:10+00:00 | v
2018-10-17 13:00:30+00:00 |
2018-10-17 13:00:30+00:00 | c
2018-10-17 13:00:50+00:00 | p
2018-10-17 13:01:00+00:00 |
2018-10-17 13:01:00+00:00 | m
2018-10-17 13:01:00+00:00 | s
2018-10-17 13:01:00+00:00 | b
Now, I would like to make a new column, that would count the number of times that the email repeated in the last 30 seconds before the entry, for example.
timestamp | email | count | comment
2018-10-17 13:00:00+00:00 | m | 1 |
2018-10-17 13:00:00+00:00 | m | 2 | (there were 2 entries in the last 30s)
2018-10-17 13:00:10+00:00 | | 1 | (empty we count as well)
2018-10-17 13:00:10+00:00 | v | 1 |
2018-10-17 13:00:30+00:00 | | 2 | (counting the empty like emails)
2018-10-17 13:00:30+00:00 | c | 1 |
2018-10-17 13:00:50+00:00 | p | 1 |
2018-10-17 13:01:00+00:00 | | 2 | (in the last 30s from this ts, we have 2)
2018-10-17 13:01:00+00:00 | m | 1 | (the first 2 m happened before the last 30s)
2018-10-17 13:01:00+00:00 | s | 1 |
2018-10-17 13:01:00+00:00 | b | 1 |
The timestamp is a dateTime object
timestamp datetime64[ns, UTC]
Also, it's the index and it's sorted.
I first tried, this command:
df['email'].groupby(df.email).rolling('120s').count().values
But it doesn't work with a string, so I converted it to a unique number, using:
full_df['email'].factorize()
But the results doesn't appear to be right:
timestamp | email | count | comment
2018-10-17 13:00:00+00:00 | m | 1 |
2018-10-17 13:00:00+00:00 | m | 2 |
2018-10-17 13:00:10+00:00 | | 1 |
2018-10-17 13:00:10+00:00 | v | 2 | (No ideia about this result)
2018-10-17 13:00:30+00:00 | | 3 | (Appears to just keeping count)
2018-10-17 13:00:30+00:00 | c | 1 | (Then just go back to 1 again... )
2018-10-17 13:00:50+00:00 | p | 2 |
2018-10-17 13:01:00+00:00 | | 3 |
2018-10-17 13:01:00+00:00 | m | 4 |
2018-10-17 13:01:00+00:00 | s | 1 |
2018-10-17 13:01:00+00:00 | b | 1 |
Any ideia what I'm doing wrong and how can I get what I would like to get?
Many Thanks,
Joao
python pandas jupyter
I have a table like this (email is simplified to just one letter here):
timestamp | email
2018-10-17 13:00:00+00:00 | m
2018-10-17 13:00:00+00:00 | m
2018-10-17 13:00:10+00:00 |
2018-10-17 13:00:10+00:00 | v
2018-10-17 13:00:30+00:00 |
2018-10-17 13:00:30+00:00 | c
2018-10-17 13:00:50+00:00 | p
2018-10-17 13:01:00+00:00 |
2018-10-17 13:01:00+00:00 | m
2018-10-17 13:01:00+00:00 | s
2018-10-17 13:01:00+00:00 | b
Now, I would like to make a new column, that would count the number of times that the email repeated in the last 30 seconds before the entry, for example.
timestamp | email | count | comment
2018-10-17 13:00:00+00:00 | m | 1 |
2018-10-17 13:00:00+00:00 | m | 2 | (there were 2 entries in the last 30s)
2018-10-17 13:00:10+00:00 | | 1 | (empty we count as well)
2018-10-17 13:00:10+00:00 | v | 1 |
2018-10-17 13:00:30+00:00 | | 2 | (counting the empty like emails)
2018-10-17 13:00:30+00:00 | c | 1 |
2018-10-17 13:00:50+00:00 | p | 1 |
2018-10-17 13:01:00+00:00 | | 2 | (in the last 30s from this ts, we have 2)
2018-10-17 13:01:00+00:00 | m | 1 | (the first 2 m happened before the last 30s)
2018-10-17 13:01:00+00:00 | s | 1 |
2018-10-17 13:01:00+00:00 | b | 1 |
The timestamp is a dateTime object
timestamp datetime64[ns, UTC]
Also, it's the index and it's sorted.
I first tried, this command:
df['email'].groupby(df.email).rolling('120s').count().values
But it doesn't work with a string, so I converted it to a unique number, using:
full_df['email'].factorize()
But the results doesn't appear to be right:
timestamp | email | count | comment
2018-10-17 13:00:00+00:00 | m | 1 |
2018-10-17 13:00:00+00:00 | m | 2 |
2018-10-17 13:00:10+00:00 | | 1 |
2018-10-17 13:00:10+00:00 | v | 2 | (No ideia about this result)
2018-10-17 13:00:30+00:00 | | 3 | (Appears to just keeping count)
2018-10-17 13:00:30+00:00 | c | 1 | (Then just go back to 1 again... )
2018-10-17 13:00:50+00:00 | p | 2 |
2018-10-17 13:01:00+00:00 | | 3 |
2018-10-17 13:01:00+00:00 | m | 4 |
2018-10-17 13:01:00+00:00 | s | 1 |
2018-10-17 13:01:00+00:00 | b | 1 |
Any ideia what I'm doing wrong and how can I get what I would like to get?
Many Thanks,
Joao
python pandas jupyter
python pandas jupyter
edited Nov 14 '18 at 17:40


yatu
9,48111031
9,48111031
asked Nov 14 '18 at 15:22
joao.sauerjoao.sauer
1249
1249
add a comment |
add a comment |
1 Answer
1
active
oldest
votes
You can use apply
after rolling
to count the number of times the last element of the window shows up in the window like this:
df['count'] = df['email'].astype('category').cat.codes.rolling('30s').apply(lambda x: sum(x==x[-1]))
Many thanks! Worked perfect!
– joao.sauer
Nov 14 '18 at 22:21
add a comment |
Your Answer
StackExchange.ifUsing("editor", function () {
StackExchange.using("externalEditor", function () {
StackExchange.using("snippets", function () {
StackExchange.snippets.init();
});
});
}, "code-snippets");
StackExchange.ready(function() {
var channelOptions = {
tags: "".split(" "),
id: "1"
};
initTagRenderer("".split(" "), "".split(" "), channelOptions);
StackExchange.using("externalEditor", function() {
// Have to fire editor after snippets, if snippets enabled
if (StackExchange.settings.snippets.snippetsEnabled) {
StackExchange.using("snippets", function() {
createEditor();
});
}
else {
createEditor();
}
});
function createEditor() {
StackExchange.prepareEditor({
heartbeatType: 'answer',
autoActivateHeartbeat: false,
convertImagesToLinks: true,
noModals: true,
showLowRepImageUploadWarning: true,
reputationToPostImages: 10,
bindNavPrevention: true,
postfix: "",
imageUploader: {
brandingHtml: "Powered by u003ca class="icon-imgur-white" href="https://imgur.com/"u003eu003c/au003e",
contentPolicyHtml: "User contributions licensed under u003ca href="https://creativecommons.org/licenses/by-sa/3.0/"u003ecc by-sa 3.0 with attribution requiredu003c/au003e u003ca href="https://stackoverflow.com/legal/content-policy"u003e(content policy)u003c/au003e",
allowUrls: true
},
onDemand: true,
discardSelector: ".discard-answer"
,immediatelyShowMarkdownHelp:true
});
}
});
Sign up or log in
StackExchange.ready(function () {
StackExchange.helpers.onClickDraftSave('#login-link');
});
Sign up using Google
Sign up using Facebook
Sign up using Email and Password
Post as a guest
Required, but never shown
StackExchange.ready(
function () {
StackExchange.openid.initPostLogin('.new-post-login', 'https%3a%2f%2fstackoverflow.com%2fquestions%2f53303490%2fpandas-count-like-a-stack-using-rolling%23new-answer', 'question_page');
}
);
Post as a guest
Required, but never shown
1 Answer
1
active
oldest
votes
1 Answer
1
active
oldest
votes
active
oldest
votes
active
oldest
votes
You can use apply
after rolling
to count the number of times the last element of the window shows up in the window like this:
df['count'] = df['email'].astype('category').cat.codes.rolling('30s').apply(lambda x: sum(x==x[-1]))
Many thanks! Worked perfect!
– joao.sauer
Nov 14 '18 at 22:21
add a comment |
You can use apply
after rolling
to count the number of times the last element of the window shows up in the window like this:
df['count'] = df['email'].astype('category').cat.codes.rolling('30s').apply(lambda x: sum(x==x[-1]))
Many thanks! Worked perfect!
– joao.sauer
Nov 14 '18 at 22:21
add a comment |
You can use apply
after rolling
to count the number of times the last element of the window shows up in the window like this:
df['count'] = df['email'].astype('category').cat.codes.rolling('30s').apply(lambda x: sum(x==x[-1]))
You can use apply
after rolling
to count the number of times the last element of the window shows up in the window like this:
df['count'] = df['email'].astype('category').cat.codes.rolling('30s').apply(lambda x: sum(x==x[-1]))
answered Nov 14 '18 at 18:12
Franco PiccoloFranco Piccolo
1,581713
1,581713
Many thanks! Worked perfect!
– joao.sauer
Nov 14 '18 at 22:21
add a comment |
Many thanks! Worked perfect!
– joao.sauer
Nov 14 '18 at 22:21
Many thanks! Worked perfect!
– joao.sauer
Nov 14 '18 at 22:21
Many thanks! Worked perfect!
– joao.sauer
Nov 14 '18 at 22:21
add a comment |
Thanks for contributing an answer to Stack Overflow!
- Please be sure to answer the question. Provide details and share your research!
But avoid …
- Asking for help, clarification, or responding to other answers.
- Making statements based on opinion; back them up with references or personal experience.
To learn more, see our tips on writing great answers.
Sign up or log in
StackExchange.ready(function () {
StackExchange.helpers.onClickDraftSave('#login-link');
});
Sign up using Google
Sign up using Facebook
Sign up using Email and Password
Post as a guest
Required, but never shown
StackExchange.ready(
function () {
StackExchange.openid.initPostLogin('.new-post-login', 'https%3a%2f%2fstackoverflow.com%2fquestions%2f53303490%2fpandas-count-like-a-stack-using-rolling%23new-answer', 'question_page');
}
);
Post as a guest
Required, but never shown
Sign up or log in
StackExchange.ready(function () {
StackExchange.helpers.onClickDraftSave('#login-link');
});
Sign up using Google
Sign up using Facebook
Sign up using Email and Password
Post as a guest
Required, but never shown
Sign up or log in
StackExchange.ready(function () {
StackExchange.helpers.onClickDraftSave('#login-link');
});
Sign up using Google
Sign up using Facebook
Sign up using Email and Password
Post as a guest
Required, but never shown
Sign up or log in
StackExchange.ready(function () {
StackExchange.helpers.onClickDraftSave('#login-link');
});
Sign up using Google
Sign up using Facebook
Sign up using Email and Password
Sign up using Google
Sign up using Facebook
Sign up using Email and Password
Post as a guest
Required, but never shown
Required, but never shown
Required, but never shown
Required, but never shown
Required, but never shown
Required, but never shown
Required, but never shown
Required, but never shown
Required, but never shown
J3Ztm8,re x3MrNcdX7