A simple explanation of Random Forest
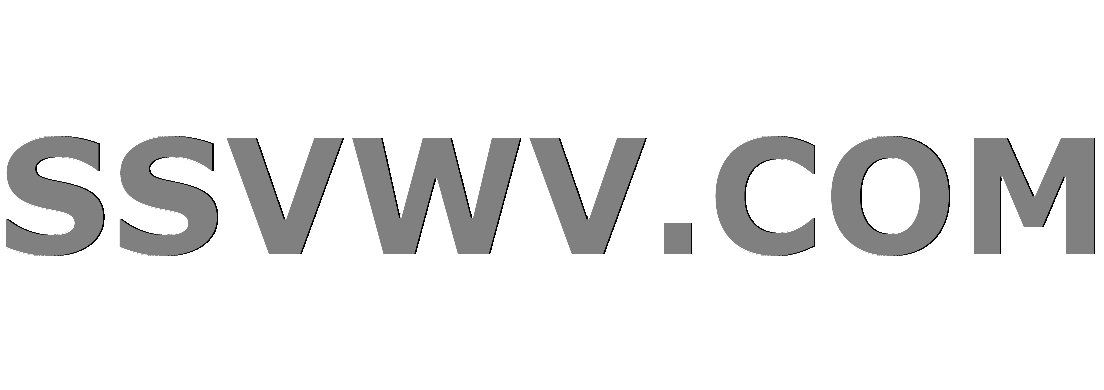
Multi tool use
up vote
6
down vote
favorite
I'm trying to understand how random forest works in plain English instead of mathematics. Can anybody give me a really simple explanation of how this algorithm works?
As far as I understand, we feed the features and labels without telling the algorithm which feature should be classified as which label? As I used to do Naive Bayes which is based on probability we need to tell which feature should be which label. Am I completely far off?
If I can get any very simple explanation I'd be really appreciated.
algorithm classification random-forest
add a comment |
up vote
6
down vote
favorite
I'm trying to understand how random forest works in plain English instead of mathematics. Can anybody give me a really simple explanation of how this algorithm works?
As far as I understand, we feed the features and labels without telling the algorithm which feature should be classified as which label? As I used to do Naive Bayes which is based on probability we need to tell which feature should be which label. Am I completely far off?
If I can get any very simple explanation I'd be really appreciated.
algorithm classification random-forest
5
quora.com/Random-Forests/…
– CoryKramer
Jul 10 '15 at 15:35
add a comment |
up vote
6
down vote
favorite
up vote
6
down vote
favorite
I'm trying to understand how random forest works in plain English instead of mathematics. Can anybody give me a really simple explanation of how this algorithm works?
As far as I understand, we feed the features and labels without telling the algorithm which feature should be classified as which label? As I used to do Naive Bayes which is based on probability we need to tell which feature should be which label. Am I completely far off?
If I can get any very simple explanation I'd be really appreciated.
algorithm classification random-forest
I'm trying to understand how random forest works in plain English instead of mathematics. Can anybody give me a really simple explanation of how this algorithm works?
As far as I understand, we feed the features and labels without telling the algorithm which feature should be classified as which label? As I used to do Naive Bayes which is based on probability we need to tell which feature should be which label. Am I completely far off?
If I can get any very simple explanation I'd be really appreciated.
algorithm classification random-forest
algorithm classification random-forest
edited Nov 11 at 5:02
bearbear123
112
112
asked Jul 10 '15 at 15:32
toy
4,4871357110
4,4871357110
5
quora.com/Random-Forests/…
– CoryKramer
Jul 10 '15 at 15:35
add a comment |
5
quora.com/Random-Forests/…
– CoryKramer
Jul 10 '15 at 15:35
5
5
quora.com/Random-Forests/…
– CoryKramer
Jul 10 '15 at 15:35
quora.com/Random-Forests/…
– CoryKramer
Jul 10 '15 at 15:35
add a comment |
3 Answers
3
active
oldest
votes
up vote
6
down vote
accepted
RandomForest
uses a so-called bagging approach. The idea is based on the classic bias-variance trade off. Suppose that we have a set (say N
) of overfitted estimators that have low bias but high cross-sample-variance. So low bias is good and we want to keep it, high variance is bad and we want to reduce it. RandomForest
tries to achieve this by doing a so-called bootstraps/sub-sampling (as @Alexander mentioned, this is a combination of bootstrap sampling on both observations and features). The prediction is the average of individual estimators so the low-bias property is successfully preserved. And further by Central Limit Theorem, the variance of this sample average has a variance equal to variance of individual estimator divided by square root of N
. So now, it has both low-bias and low-variance properties, and this is why RandomForest
often outperforms stand-alone estimator.
To slightly extend Jianxun's excellent summary above, a RandomForest typically takes a random selection of one-third of the attributes at each node in the tree for a regression problem (and the square root of the number of attributes for a classification problem). So it is a combination of bagging (taking random bootstrap samples of the original data) and random attribute selection.
– Alexander
Jul 11 '15 at 16:35
add a comment |
up vote
8
down vote
I will try to give another complementary explanation with simple words.
A random forest is a collection of random decision trees (of number n_estimators
in sklearn).
What you need to understand is how to build one random decision tree.
Roughly speaking, to build a random decision tree you start from a subset of your training samples. At each node you will draw randomly a subset of features (number determined by max_features
in sklearn). For each of these features you will test different thresholds and see how they split your samples according to a given criterion (generally entropy or gini, criterion
parameter in sklearn). Then you will keep the feature and its threshold that best split your data and record it in the node.
When the construction of the tree ends (it can be for different reasons: maximum depth is reached (max_depth
in sklearn), minimum sample number is reached (min_samples_leaf
in sklearn) etc.) you look at the samples in each leaf and keep the frequency of the labels.
As a result, it is like the tree gives you a partition of your training samples according to meaningful features.
As each node is built from features taken randomly, you understand that each tree built in this way will be different. This contributes to the good compromise between bias and variance, as explained by @Jianxun Li.
Then in testing mode, a test sample will go through each tree, giving you label frequencies for each tree. The most represented label is generally the final classification result.
add a comment |
up vote
7
down vote
Adding on to the above two answers, Since you mentioned a simple explanation. Here is a write up that I feel is the most simple way you can explain random forests.
Credits go to Edwin Chen for the simple explanation here in layman terms for random forests. Posting the same below.
Suppose you’re very indecisive, so whenever you want to watch a movie, you ask your friend Willow if she thinks you’ll like it. In order to answer, Willow first needs to figure out what movies you like, so you give her a bunch of movies and tell her whether you liked each one or not (i.e., you give her a labeled training set). Then, when you ask her if she thinks you’ll like movie X or not, she plays a 20 questions-like game with IMDB, asking questions like “Is X a romantic movie?”, “Does Johnny Depp star in X?”, and so on. She asks more informative questions first (i.e., she maximizes the information gain of each question), and gives you a yes/no answer at the end.
Thus, Willow is a decision tree for your movie preferences.
But Willow is only human, so she doesn’t always generalize your preferences very well (i.e., she overfits). In order to get more accurate recommendations, you’d like to ask a bunch of your friends and watch movie X if most of them say they think you’ll like it. That is, instead of asking only Willow, you want to ask Woody, Apple, and Cartman as well, and they vote on whether you’ll like a movie (i.e., you build an ensemble classifier, aka a forest in this case).
Now you don’t want each of your friends to do the same thing and give you the same answer, so you first give each of them slightly different data. After all, you’re not absolutely sure of your preferences yourself – you told Willow you loved Titanic, but maybe you were just happy that day because it was your birthday, so maybe some of your friends shouldn’t use the fact that you liked Titanic in making their recommendations. Or maybe you told her you loved Cinderella, but actually you really really loved it, so some of your friends should give Cinderella more weight. So instead of giving your friends the same data you gave Willow, you give them slightly perturbed versions. You don’t change your love/hate decisions, you just say you love/hate some movies a little more or less (formally, you give each of your friends a bootstrapped version of your original training data). For example, whereas you told Willow that you liked Black Swan and Harry Potter and disliked Avatar, you tell Woody that you liked Black Swan so much you watched it twice, you disliked Avatar, and don’t mention Harry Potter at all.
By using this ensemble, you hope that while each of your friends gives somewhat idiosyncratic recommendations (Willow thinks you like vampire movies more than you do, Woody thinks you like Pixar movies, and Cartman thinks you just hate everything), the errors get canceled out in the majority. Thus, your friends now form a bagged (bootstrap aggregated) forest of your movie preferences.
There’s still one problem with your data, however. While you loved both Titanic and Inception, it wasn’t because you like movies that star Leonardo DiCaprio. Maybe you liked both movies for other reasons. Thus, you don’t want your friends to all base their recommendations on whether Leo is in a movie or not. So when each friend asks IMDB a question, only a random subset of the possible questions is allowed (i.e., when you’re building a decision tree, at each node you use some randomness in selecting the attribute to split on, say by randomly selecting an attribute or by selecting an attribute from a random subset). This means your friends aren’t allowed to ask whether Leonardo DiCaprio is in the movie whenever they want. So whereas previously you injected randomness at the data level, by perturbing your movie preferences slightly, now you’re injecting randomness at the model level, by making your friends ask different questions at different times.
And so your friends now form a random forest.
add a comment |
3 Answers
3
active
oldest
votes
3 Answers
3
active
oldest
votes
active
oldest
votes
active
oldest
votes
up vote
6
down vote
accepted
RandomForest
uses a so-called bagging approach. The idea is based on the classic bias-variance trade off. Suppose that we have a set (say N
) of overfitted estimators that have low bias but high cross-sample-variance. So low bias is good and we want to keep it, high variance is bad and we want to reduce it. RandomForest
tries to achieve this by doing a so-called bootstraps/sub-sampling (as @Alexander mentioned, this is a combination of bootstrap sampling on both observations and features). The prediction is the average of individual estimators so the low-bias property is successfully preserved. And further by Central Limit Theorem, the variance of this sample average has a variance equal to variance of individual estimator divided by square root of N
. So now, it has both low-bias and low-variance properties, and this is why RandomForest
often outperforms stand-alone estimator.
To slightly extend Jianxun's excellent summary above, a RandomForest typically takes a random selection of one-third of the attributes at each node in the tree for a regression problem (and the square root of the number of attributes for a classification problem). So it is a combination of bagging (taking random bootstrap samples of the original data) and random attribute selection.
– Alexander
Jul 11 '15 at 16:35
add a comment |
up vote
6
down vote
accepted
RandomForest
uses a so-called bagging approach. The idea is based on the classic bias-variance trade off. Suppose that we have a set (say N
) of overfitted estimators that have low bias but high cross-sample-variance. So low bias is good and we want to keep it, high variance is bad and we want to reduce it. RandomForest
tries to achieve this by doing a so-called bootstraps/sub-sampling (as @Alexander mentioned, this is a combination of bootstrap sampling on both observations and features). The prediction is the average of individual estimators so the low-bias property is successfully preserved. And further by Central Limit Theorem, the variance of this sample average has a variance equal to variance of individual estimator divided by square root of N
. So now, it has both low-bias and low-variance properties, and this is why RandomForest
often outperforms stand-alone estimator.
To slightly extend Jianxun's excellent summary above, a RandomForest typically takes a random selection of one-third of the attributes at each node in the tree for a regression problem (and the square root of the number of attributes for a classification problem). So it is a combination of bagging (taking random bootstrap samples of the original data) and random attribute selection.
– Alexander
Jul 11 '15 at 16:35
add a comment |
up vote
6
down vote
accepted
up vote
6
down vote
accepted
RandomForest
uses a so-called bagging approach. The idea is based on the classic bias-variance trade off. Suppose that we have a set (say N
) of overfitted estimators that have low bias but high cross-sample-variance. So low bias is good and we want to keep it, high variance is bad and we want to reduce it. RandomForest
tries to achieve this by doing a so-called bootstraps/sub-sampling (as @Alexander mentioned, this is a combination of bootstrap sampling on both observations and features). The prediction is the average of individual estimators so the low-bias property is successfully preserved. And further by Central Limit Theorem, the variance of this sample average has a variance equal to variance of individual estimator divided by square root of N
. So now, it has both low-bias and low-variance properties, and this is why RandomForest
often outperforms stand-alone estimator.
RandomForest
uses a so-called bagging approach. The idea is based on the classic bias-variance trade off. Suppose that we have a set (say N
) of overfitted estimators that have low bias but high cross-sample-variance. So low bias is good and we want to keep it, high variance is bad and we want to reduce it. RandomForest
tries to achieve this by doing a so-called bootstraps/sub-sampling (as @Alexander mentioned, this is a combination of bootstrap sampling on both observations and features). The prediction is the average of individual estimators so the low-bias property is successfully preserved. And further by Central Limit Theorem, the variance of this sample average has a variance equal to variance of individual estimator divided by square root of N
. So now, it has both low-bias and low-variance properties, and this is why RandomForest
often outperforms stand-alone estimator.
edited Jul 11 '15 at 20:04
answered Jul 10 '15 at 15:42


Jianxun Li
13.9k23052
13.9k23052
To slightly extend Jianxun's excellent summary above, a RandomForest typically takes a random selection of one-third of the attributes at each node in the tree for a regression problem (and the square root of the number of attributes for a classification problem). So it is a combination of bagging (taking random bootstrap samples of the original data) and random attribute selection.
– Alexander
Jul 11 '15 at 16:35
add a comment |
To slightly extend Jianxun's excellent summary above, a RandomForest typically takes a random selection of one-third of the attributes at each node in the tree for a regression problem (and the square root of the number of attributes for a classification problem). So it is a combination of bagging (taking random bootstrap samples of the original data) and random attribute selection.
– Alexander
Jul 11 '15 at 16:35
To slightly extend Jianxun's excellent summary above, a RandomForest typically takes a random selection of one-third of the attributes at each node in the tree for a regression problem (and the square root of the number of attributes for a classification problem). So it is a combination of bagging (taking random bootstrap samples of the original data) and random attribute selection.
– Alexander
Jul 11 '15 at 16:35
To slightly extend Jianxun's excellent summary above, a RandomForest typically takes a random selection of one-third of the attributes at each node in the tree for a regression problem (and the square root of the number of attributes for a classification problem). So it is a combination of bagging (taking random bootstrap samples of the original data) and random attribute selection.
– Alexander
Jul 11 '15 at 16:35
add a comment |
up vote
8
down vote
I will try to give another complementary explanation with simple words.
A random forest is a collection of random decision trees (of number n_estimators
in sklearn).
What you need to understand is how to build one random decision tree.
Roughly speaking, to build a random decision tree you start from a subset of your training samples. At each node you will draw randomly a subset of features (number determined by max_features
in sklearn). For each of these features you will test different thresholds and see how they split your samples according to a given criterion (generally entropy or gini, criterion
parameter in sklearn). Then you will keep the feature and its threshold that best split your data and record it in the node.
When the construction of the tree ends (it can be for different reasons: maximum depth is reached (max_depth
in sklearn), minimum sample number is reached (min_samples_leaf
in sklearn) etc.) you look at the samples in each leaf and keep the frequency of the labels.
As a result, it is like the tree gives you a partition of your training samples according to meaningful features.
As each node is built from features taken randomly, you understand that each tree built in this way will be different. This contributes to the good compromise between bias and variance, as explained by @Jianxun Li.
Then in testing mode, a test sample will go through each tree, giving you label frequencies for each tree. The most represented label is generally the final classification result.
add a comment |
up vote
8
down vote
I will try to give another complementary explanation with simple words.
A random forest is a collection of random decision trees (of number n_estimators
in sklearn).
What you need to understand is how to build one random decision tree.
Roughly speaking, to build a random decision tree you start from a subset of your training samples. At each node you will draw randomly a subset of features (number determined by max_features
in sklearn). For each of these features you will test different thresholds and see how they split your samples according to a given criterion (generally entropy or gini, criterion
parameter in sklearn). Then you will keep the feature and its threshold that best split your data and record it in the node.
When the construction of the tree ends (it can be for different reasons: maximum depth is reached (max_depth
in sklearn), minimum sample number is reached (min_samples_leaf
in sklearn) etc.) you look at the samples in each leaf and keep the frequency of the labels.
As a result, it is like the tree gives you a partition of your training samples according to meaningful features.
As each node is built from features taken randomly, you understand that each tree built in this way will be different. This contributes to the good compromise between bias and variance, as explained by @Jianxun Li.
Then in testing mode, a test sample will go through each tree, giving you label frequencies for each tree. The most represented label is generally the final classification result.
add a comment |
up vote
8
down vote
up vote
8
down vote
I will try to give another complementary explanation with simple words.
A random forest is a collection of random decision trees (of number n_estimators
in sklearn).
What you need to understand is how to build one random decision tree.
Roughly speaking, to build a random decision tree you start from a subset of your training samples. At each node you will draw randomly a subset of features (number determined by max_features
in sklearn). For each of these features you will test different thresholds and see how they split your samples according to a given criterion (generally entropy or gini, criterion
parameter in sklearn). Then you will keep the feature and its threshold that best split your data and record it in the node.
When the construction of the tree ends (it can be for different reasons: maximum depth is reached (max_depth
in sklearn), minimum sample number is reached (min_samples_leaf
in sklearn) etc.) you look at the samples in each leaf and keep the frequency of the labels.
As a result, it is like the tree gives you a partition of your training samples according to meaningful features.
As each node is built from features taken randomly, you understand that each tree built in this way will be different. This contributes to the good compromise between bias and variance, as explained by @Jianxun Li.
Then in testing mode, a test sample will go through each tree, giving you label frequencies for each tree. The most represented label is generally the final classification result.
I will try to give another complementary explanation with simple words.
A random forest is a collection of random decision trees (of number n_estimators
in sklearn).
What you need to understand is how to build one random decision tree.
Roughly speaking, to build a random decision tree you start from a subset of your training samples. At each node you will draw randomly a subset of features (number determined by max_features
in sklearn). For each of these features you will test different thresholds and see how they split your samples according to a given criterion (generally entropy or gini, criterion
parameter in sklearn). Then you will keep the feature and its threshold that best split your data and record it in the node.
When the construction of the tree ends (it can be for different reasons: maximum depth is reached (max_depth
in sklearn), minimum sample number is reached (min_samples_leaf
in sklearn) etc.) you look at the samples in each leaf and keep the frequency of the labels.
As a result, it is like the tree gives you a partition of your training samples according to meaningful features.
As each node is built from features taken randomly, you understand that each tree built in this way will be different. This contributes to the good compromise between bias and variance, as explained by @Jianxun Li.
Then in testing mode, a test sample will go through each tree, giving you label frequencies for each tree. The most represented label is generally the final classification result.
answered Aug 20 '15 at 13:18
RomaneG
1956
1956
add a comment |
add a comment |
up vote
7
down vote
Adding on to the above two answers, Since you mentioned a simple explanation. Here is a write up that I feel is the most simple way you can explain random forests.
Credits go to Edwin Chen for the simple explanation here in layman terms for random forests. Posting the same below.
Suppose you’re very indecisive, so whenever you want to watch a movie, you ask your friend Willow if she thinks you’ll like it. In order to answer, Willow first needs to figure out what movies you like, so you give her a bunch of movies and tell her whether you liked each one or not (i.e., you give her a labeled training set). Then, when you ask her if she thinks you’ll like movie X or not, she plays a 20 questions-like game with IMDB, asking questions like “Is X a romantic movie?”, “Does Johnny Depp star in X?”, and so on. She asks more informative questions first (i.e., she maximizes the information gain of each question), and gives you a yes/no answer at the end.
Thus, Willow is a decision tree for your movie preferences.
But Willow is only human, so she doesn’t always generalize your preferences very well (i.e., she overfits). In order to get more accurate recommendations, you’d like to ask a bunch of your friends and watch movie X if most of them say they think you’ll like it. That is, instead of asking only Willow, you want to ask Woody, Apple, and Cartman as well, and they vote on whether you’ll like a movie (i.e., you build an ensemble classifier, aka a forest in this case).
Now you don’t want each of your friends to do the same thing and give you the same answer, so you first give each of them slightly different data. After all, you’re not absolutely sure of your preferences yourself – you told Willow you loved Titanic, but maybe you were just happy that day because it was your birthday, so maybe some of your friends shouldn’t use the fact that you liked Titanic in making their recommendations. Or maybe you told her you loved Cinderella, but actually you really really loved it, so some of your friends should give Cinderella more weight. So instead of giving your friends the same data you gave Willow, you give them slightly perturbed versions. You don’t change your love/hate decisions, you just say you love/hate some movies a little more or less (formally, you give each of your friends a bootstrapped version of your original training data). For example, whereas you told Willow that you liked Black Swan and Harry Potter and disliked Avatar, you tell Woody that you liked Black Swan so much you watched it twice, you disliked Avatar, and don’t mention Harry Potter at all.
By using this ensemble, you hope that while each of your friends gives somewhat idiosyncratic recommendations (Willow thinks you like vampire movies more than you do, Woody thinks you like Pixar movies, and Cartman thinks you just hate everything), the errors get canceled out in the majority. Thus, your friends now form a bagged (bootstrap aggregated) forest of your movie preferences.
There’s still one problem with your data, however. While you loved both Titanic and Inception, it wasn’t because you like movies that star Leonardo DiCaprio. Maybe you liked both movies for other reasons. Thus, you don’t want your friends to all base their recommendations on whether Leo is in a movie or not. So when each friend asks IMDB a question, only a random subset of the possible questions is allowed (i.e., when you’re building a decision tree, at each node you use some randomness in selecting the attribute to split on, say by randomly selecting an attribute or by selecting an attribute from a random subset). This means your friends aren’t allowed to ask whether Leonardo DiCaprio is in the movie whenever they want. So whereas previously you injected randomness at the data level, by perturbing your movie preferences slightly, now you’re injecting randomness at the model level, by making your friends ask different questions at different times.
And so your friends now form a random forest.
add a comment |
up vote
7
down vote
Adding on to the above two answers, Since you mentioned a simple explanation. Here is a write up that I feel is the most simple way you can explain random forests.
Credits go to Edwin Chen for the simple explanation here in layman terms for random forests. Posting the same below.
Suppose you’re very indecisive, so whenever you want to watch a movie, you ask your friend Willow if she thinks you’ll like it. In order to answer, Willow first needs to figure out what movies you like, so you give her a bunch of movies and tell her whether you liked each one or not (i.e., you give her a labeled training set). Then, when you ask her if she thinks you’ll like movie X or not, she plays a 20 questions-like game with IMDB, asking questions like “Is X a romantic movie?”, “Does Johnny Depp star in X?”, and so on. She asks more informative questions first (i.e., she maximizes the information gain of each question), and gives you a yes/no answer at the end.
Thus, Willow is a decision tree for your movie preferences.
But Willow is only human, so she doesn’t always generalize your preferences very well (i.e., she overfits). In order to get more accurate recommendations, you’d like to ask a bunch of your friends and watch movie X if most of them say they think you’ll like it. That is, instead of asking only Willow, you want to ask Woody, Apple, and Cartman as well, and they vote on whether you’ll like a movie (i.e., you build an ensemble classifier, aka a forest in this case).
Now you don’t want each of your friends to do the same thing and give you the same answer, so you first give each of them slightly different data. After all, you’re not absolutely sure of your preferences yourself – you told Willow you loved Titanic, but maybe you were just happy that day because it was your birthday, so maybe some of your friends shouldn’t use the fact that you liked Titanic in making their recommendations. Or maybe you told her you loved Cinderella, but actually you really really loved it, so some of your friends should give Cinderella more weight. So instead of giving your friends the same data you gave Willow, you give them slightly perturbed versions. You don’t change your love/hate decisions, you just say you love/hate some movies a little more or less (formally, you give each of your friends a bootstrapped version of your original training data). For example, whereas you told Willow that you liked Black Swan and Harry Potter and disliked Avatar, you tell Woody that you liked Black Swan so much you watched it twice, you disliked Avatar, and don’t mention Harry Potter at all.
By using this ensemble, you hope that while each of your friends gives somewhat idiosyncratic recommendations (Willow thinks you like vampire movies more than you do, Woody thinks you like Pixar movies, and Cartman thinks you just hate everything), the errors get canceled out in the majority. Thus, your friends now form a bagged (bootstrap aggregated) forest of your movie preferences.
There’s still one problem with your data, however. While you loved both Titanic and Inception, it wasn’t because you like movies that star Leonardo DiCaprio. Maybe you liked both movies for other reasons. Thus, you don’t want your friends to all base their recommendations on whether Leo is in a movie or not. So when each friend asks IMDB a question, only a random subset of the possible questions is allowed (i.e., when you’re building a decision tree, at each node you use some randomness in selecting the attribute to split on, say by randomly selecting an attribute or by selecting an attribute from a random subset). This means your friends aren’t allowed to ask whether Leonardo DiCaprio is in the movie whenever they want. So whereas previously you injected randomness at the data level, by perturbing your movie preferences slightly, now you’re injecting randomness at the model level, by making your friends ask different questions at different times.
And so your friends now form a random forest.
add a comment |
up vote
7
down vote
up vote
7
down vote
Adding on to the above two answers, Since you mentioned a simple explanation. Here is a write up that I feel is the most simple way you can explain random forests.
Credits go to Edwin Chen for the simple explanation here in layman terms for random forests. Posting the same below.
Suppose you’re very indecisive, so whenever you want to watch a movie, you ask your friend Willow if she thinks you’ll like it. In order to answer, Willow first needs to figure out what movies you like, so you give her a bunch of movies and tell her whether you liked each one or not (i.e., you give her a labeled training set). Then, when you ask her if she thinks you’ll like movie X or not, she plays a 20 questions-like game with IMDB, asking questions like “Is X a romantic movie?”, “Does Johnny Depp star in X?”, and so on. She asks more informative questions first (i.e., she maximizes the information gain of each question), and gives you a yes/no answer at the end.
Thus, Willow is a decision tree for your movie preferences.
But Willow is only human, so she doesn’t always generalize your preferences very well (i.e., she overfits). In order to get more accurate recommendations, you’d like to ask a bunch of your friends and watch movie X if most of them say they think you’ll like it. That is, instead of asking only Willow, you want to ask Woody, Apple, and Cartman as well, and they vote on whether you’ll like a movie (i.e., you build an ensemble classifier, aka a forest in this case).
Now you don’t want each of your friends to do the same thing and give you the same answer, so you first give each of them slightly different data. After all, you’re not absolutely sure of your preferences yourself – you told Willow you loved Titanic, but maybe you were just happy that day because it was your birthday, so maybe some of your friends shouldn’t use the fact that you liked Titanic in making their recommendations. Or maybe you told her you loved Cinderella, but actually you really really loved it, so some of your friends should give Cinderella more weight. So instead of giving your friends the same data you gave Willow, you give them slightly perturbed versions. You don’t change your love/hate decisions, you just say you love/hate some movies a little more or less (formally, you give each of your friends a bootstrapped version of your original training data). For example, whereas you told Willow that you liked Black Swan and Harry Potter and disliked Avatar, you tell Woody that you liked Black Swan so much you watched it twice, you disliked Avatar, and don’t mention Harry Potter at all.
By using this ensemble, you hope that while each of your friends gives somewhat idiosyncratic recommendations (Willow thinks you like vampire movies more than you do, Woody thinks you like Pixar movies, and Cartman thinks you just hate everything), the errors get canceled out in the majority. Thus, your friends now form a bagged (bootstrap aggregated) forest of your movie preferences.
There’s still one problem with your data, however. While you loved both Titanic and Inception, it wasn’t because you like movies that star Leonardo DiCaprio. Maybe you liked both movies for other reasons. Thus, you don’t want your friends to all base their recommendations on whether Leo is in a movie or not. So when each friend asks IMDB a question, only a random subset of the possible questions is allowed (i.e., when you’re building a decision tree, at each node you use some randomness in selecting the attribute to split on, say by randomly selecting an attribute or by selecting an attribute from a random subset). This means your friends aren’t allowed to ask whether Leonardo DiCaprio is in the movie whenever they want. So whereas previously you injected randomness at the data level, by perturbing your movie preferences slightly, now you’re injecting randomness at the model level, by making your friends ask different questions at different times.
And so your friends now form a random forest.
Adding on to the above two answers, Since you mentioned a simple explanation. Here is a write up that I feel is the most simple way you can explain random forests.
Credits go to Edwin Chen for the simple explanation here in layman terms for random forests. Posting the same below.
Suppose you’re very indecisive, so whenever you want to watch a movie, you ask your friend Willow if she thinks you’ll like it. In order to answer, Willow first needs to figure out what movies you like, so you give her a bunch of movies and tell her whether you liked each one or not (i.e., you give her a labeled training set). Then, when you ask her if she thinks you’ll like movie X or not, she plays a 20 questions-like game with IMDB, asking questions like “Is X a romantic movie?”, “Does Johnny Depp star in X?”, and so on. She asks more informative questions first (i.e., she maximizes the information gain of each question), and gives you a yes/no answer at the end.
Thus, Willow is a decision tree for your movie preferences.
But Willow is only human, so she doesn’t always generalize your preferences very well (i.e., she overfits). In order to get more accurate recommendations, you’d like to ask a bunch of your friends and watch movie X if most of them say they think you’ll like it. That is, instead of asking only Willow, you want to ask Woody, Apple, and Cartman as well, and they vote on whether you’ll like a movie (i.e., you build an ensemble classifier, aka a forest in this case).
Now you don’t want each of your friends to do the same thing and give you the same answer, so you first give each of them slightly different data. After all, you’re not absolutely sure of your preferences yourself – you told Willow you loved Titanic, but maybe you were just happy that day because it was your birthday, so maybe some of your friends shouldn’t use the fact that you liked Titanic in making their recommendations. Or maybe you told her you loved Cinderella, but actually you really really loved it, so some of your friends should give Cinderella more weight. So instead of giving your friends the same data you gave Willow, you give them slightly perturbed versions. You don’t change your love/hate decisions, you just say you love/hate some movies a little more or less (formally, you give each of your friends a bootstrapped version of your original training data). For example, whereas you told Willow that you liked Black Swan and Harry Potter and disliked Avatar, you tell Woody that you liked Black Swan so much you watched it twice, you disliked Avatar, and don’t mention Harry Potter at all.
By using this ensemble, you hope that while each of your friends gives somewhat idiosyncratic recommendations (Willow thinks you like vampire movies more than you do, Woody thinks you like Pixar movies, and Cartman thinks you just hate everything), the errors get canceled out in the majority. Thus, your friends now form a bagged (bootstrap aggregated) forest of your movie preferences.
There’s still one problem with your data, however. While you loved both Titanic and Inception, it wasn’t because you like movies that star Leonardo DiCaprio. Maybe you liked both movies for other reasons. Thus, you don’t want your friends to all base their recommendations on whether Leo is in a movie or not. So when each friend asks IMDB a question, only a random subset of the possible questions is allowed (i.e., when you’re building a decision tree, at each node you use some randomness in selecting the attribute to split on, say by randomly selecting an attribute or by selecting an attribute from a random subset). This means your friends aren’t allowed to ask whether Leonardo DiCaprio is in the movie whenever they want. So whereas previously you injected randomness at the data level, by perturbing your movie preferences slightly, now you’re injecting randomness at the model level, by making your friends ask different questions at different times.
And so your friends now form a random forest.
answered Feb 8 '17 at 3:05


shinz4u
8017
8017
add a comment |
add a comment |
Sign up or log in
StackExchange.ready(function () {
StackExchange.helpers.onClickDraftSave('#login-link');
});
Sign up using Google
Sign up using Facebook
Sign up using Email and Password
Post as a guest
Required, but never shown
StackExchange.ready(
function () {
StackExchange.openid.initPostLogin('.new-post-login', 'https%3a%2f%2fstackoverflow.com%2fquestions%2f31344732%2fa-simple-explanation-of-random-forest%23new-answer', 'question_page');
}
);
Post as a guest
Required, but never shown
Sign up or log in
StackExchange.ready(function () {
StackExchange.helpers.onClickDraftSave('#login-link');
});
Sign up using Google
Sign up using Facebook
Sign up using Email and Password
Post as a guest
Required, but never shown
Sign up or log in
StackExchange.ready(function () {
StackExchange.helpers.onClickDraftSave('#login-link');
});
Sign up using Google
Sign up using Facebook
Sign up using Email and Password
Post as a guest
Required, but never shown
Sign up or log in
StackExchange.ready(function () {
StackExchange.helpers.onClickDraftSave('#login-link');
});
Sign up using Google
Sign up using Facebook
Sign up using Email and Password
Sign up using Google
Sign up using Facebook
Sign up using Email and Password
Post as a guest
Required, but never shown
Required, but never shown
Required, but never shown
Required, but never shown
Required, but never shown
Required, but never shown
Required, but never shown
Required, but never shown
Required, but never shown
8SD40Gke86oA gO,mgCBGyb9UBhTDGfCx5bzme7Zx0S0aQd0OuUFhgOCfbvx0TVUV
5
quora.com/Random-Forests/…
– CoryKramer
Jul 10 '15 at 15:35